One of a series of posts I will publish leading up to the Mobile World Congress (MWC). For our video overview from last year’s event, see the end of this post.
One day, Alexa might be able to understand when you’re cooking based on the sounds you make in the kitchen.
7 February 2020 (Sliema, Malta) – Take a moment to listen to the world around you. Maybe you are listening to a podcast or the sounds of office life filtered through noise-canceling headphones. Or perhaps you’re on a train or lulled by the sound of a dishwasher. Our brains are constantly taking in the sounds around us and giving us useful information.
In the coming few years, computers will begin to also process those noises to understand what’s happening around them to modify the environment, improve hearing, and notify us if something is wrong. Much like computer vision was the success story of the last decade in machine learning, the coming one will see computers gain a sense of hearing.
Ah, the possibilities:
• Last year Amazon announced Guard, a feature of the Amazon Echo devices that listens for and recognizes the sound of windows breaking.
• This year we should start seeing over-the-counter hearing aids thanks to a law passed in the U.S. in 2017.
Those devices will take advantage of noise sensing to get a feel for the environment in which someone is having a conversation and adapt to make the human voice easier to understand. A few years ago I wrote about a Carnegie Melon researcher who proposed a multi-sensor device that included noise detection to understand appliance use as a way to figure out what someone was doing in the home.
But it’s about to get a lot better. Or scarier. Or weirder. Depends on your point of view. There will be a large machine learning component at MWC this year (educational sessions, hand-on-training and vendor presentations) and one company I have my eye on is Audio Analytic which has built 700 different sound profiles that can detect everything from a train station to a baby’s cry. In addition to building and licensing sound models for companies that include Qualcomm and smart lighting company Sengeled, it has built a polyphonic sound detection score that will help others building sound detection models measure how effective their approaches are.
Note: one of the cooler events to attend is the TinyML conference which runs next week. “Tiny machine learning” is broadly defined as a fast growing field of machine learning technologies and applications including hardware (dedicated integrated circuits), algorithms and software capable of performing on-device sensor (vision, audio, IMU, biomedical, etc.) data analytics at extremely low power, typically in the mW range and below, and hence enabling a variety of always-on use-cases and targeting battery operated devices. The TinyML conference brings learning experts from the industry, academia, start-ups and government labs from all over the globe to share the “latest & greatest” in the field.
The machine learning community has robust data sets and ways to measure the effectiveness of speech and image recognition thanks to years of work and a clear understanding of what metrics matter in determining the effectiveness of an algorithm. In speech, for example, we look at the word error rate, or how often the computer messes up the word we said.
Classifying sounds is trickier. There’s no limit on the sounds that can be made in the real world, as opposed to the natural limitations of a human voice box. Researchers also have to contend with an almost infinite set of potential noises having a meaning. In language, there are a limited set of phonemes that can help shape a model, but the sounds of a mosquito, glass breaking, a refrigerator running, or a dog barking follow no common patterns.
Thus there are ways sound detection can go wrong that are familiar to anyone building a machine learning model and ways unique to the problem of recognizing millions of sounds. Having a score can help see how close a model comes. This will help the industry move toward a standard.
That standard will help push sound detection and recognition into more places. For example, at the TinyML conference Audio Analytic is showing off sound detection on a board running an ARM Cortex-M0+ processor. This is a tiny, tiny chip used for sensors. The models are shrunk down to a few kilobytes. This means we could have a small, battery-powered sensor on a wall that can detect the sound of glass breaking or a pair of headphones might come on the market that can “listen” for the sound of an approaching car and reduce their active noise cancellation so a jogger or biker could hear what’s coming.
The future of sound detection making waves breaks out into four different areas:
1. The first is in safety and security, and it’s already here in Amazon’s Guard or smart products trained to listen for the sound of smoke detectors and let someone know when they are activated.
2. The second area is in health and well being. Here we’ll find sensors trained to hear the sound of a baby’s cry, to detect coughing, or even to figure out if someone is snoring.
3. Another area (well, two areas but I combined them since they are related) are the detection of external environments for communications, as is needed for hearing aids, and the detection of external environments to improve the delivery of entertainment. As an example of how entertainment could take advantage of better sound detection, Google Nest Hub Max speakers already adjust the sound of music based on the dimensions of the room, but what if the speaker could also detect if you’re running a fan or there’s a video game playing, and adapts accordingly?
4. Environmental sound cues also play into the final area of value creation — convenience. Sound can help computers derive context that could influence how a smart home responds. For example, if a computer could recognize a set of sounds as highly correlated to cooking, it might brighten the lights in the kitchen.
The possibilities are endless, and by focusing on creating a good metric for building accurate sound detection and by bringing the technology to low-power and contained processors, we’ll have more options for the industry to play with going forward. In the next decade giving computers better hearing will mean smarter homes, better hearing aids, and a better experience for us as we navigate an increasingly loud and confusing world.
Now, our short video blast from last year’s MWC:
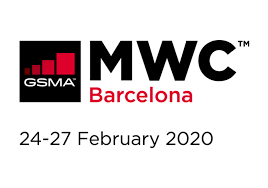